When it comes to card-not-present transactions, security is constantly a moving target. Between February and April, the peak period when COVID-19 was spreading across much of the US, cyber-attacks against the financial sector were reported to have risen by 238%.
The exponential growth of digital payment transactions, combined with the increasing variety of customer-facing devices and payment applications, has many financial institutions re-evaluating their approaches to cybersecurity. Faster fraudsters and new crime syndicates are leaving customers vulnerable, and many banks heading for a payment fraud train wreck.
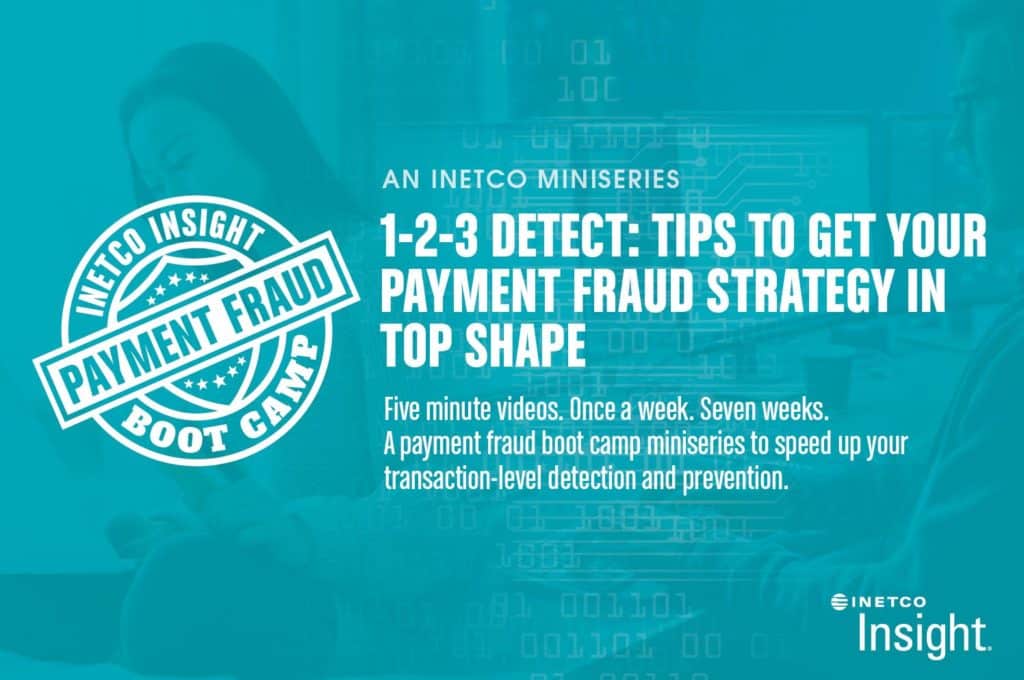
Avoiding the payment fraud train wreck
Common card-not-present attacks, such as account takeovers, SIM swaps, botnet attacks, identity testing, malware and chargebacks, need to be identified, investigated and blocked as quickly as possible – without the risk of increased false positives and unnecessary customer service (and revenue) disruption.
This is where a hybrid approach featuring the right combination of rules-based alerting, network monitoring and machine learning becomes important. Combining these features with a continuous feed of transaction data and device intelligence will put financial institutions in a position to make preemptive decisions around card-not-present fraud.
Focusing in on the right data, at the right time
Having more data points available means you can make more precise decisions at the right time. You can limit the number of false transaction rejections and take decisive action on those transactions that are fraudulent. Existing card-not-present fraud defences are strengthened through the ability to:
- Screen every transaction field in the message payload — to detect suspicious fields and respond to anomalous transaction activity in milliseconds
- Incorporate real-time machine learning that utilizes more detailed transaction data feature sets — to predict and adapt to existing and new fraud patterns that are emerging in the card-not-present space
- Feed risk scoring engines with a much broader data set — to increase the precision of transaction scoring and become more accurate at blocking and preventing fraudulent card-not-present transactions
CNP fraud prevention is crucial in today’s digital landscape to ensure secure transactions and protect both financial institutions and customers.
To learn more about the importance of screening every field of every card-not-present transaction in real-time, and more on our payment fraud strategy, take 5 minutes and listen to INETCO’s CTO, Ugan Naidoo, featured in the second episode of the 1-2-3 Detect Payment Fraud Miniseries.