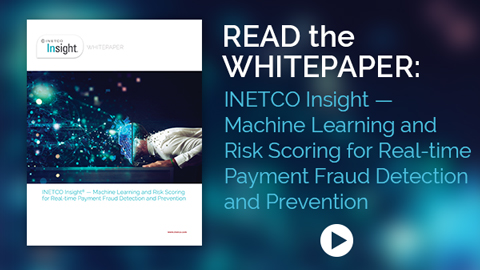
There are scary villains out there, and they don’t just come out on Halloween. Forget about tricks, and treat yourself to something worth reading…but be warned…this blog is not for the faint of heart…
The authentication or the decline of a payment transaction is often the defining factor of end customer experience. Yet risk scoring for card present and card-not-present (CNP) transactions is still notoriously inaccurate, often failing to detect high-risk customers while generating thousands of false positives. Here are a few tips that can help improve your ability to quickly identify suspect transactions, and approve or decline them correctly.
Tip #1: Make the move towards consistent data acquisition across all payments channels
Poor data quality is the single biggest contributor to the inaccuracy of risk scoring models. This is because efficient harnessing of data across disparate data stores, multiple channels and different update frequencies has reached a near-impossible task. Implementing an independent solution designed to capture and decode data across every link of a payment transaction journey will result in optimized data acquisition and easy access to all the message fields needed to improve the precision of rules-based alerting and real-time risk scoring. This in turn means fewer legitimate customers accidentally blocked from accounts.
Tip #2: Think real-time, all the time
Effectively managing the service and fraud security aspects of every real-time payment requires continuous assessment of how customers are behaving, and whether all pieces of the real-time payments chain are working and responding as expected. The ability to examine transactions in milliseconds, rebuild individual customer risk models on the fly and extract anomalous behavioral patterns based on past card transactions will have a huge impact on how fast and accurately you can detect payment fraud.
This is why it is time to consider replacing existing approaches that only rebuild customer models as part of a scheduled batch ETL process with continuously evolving solutions that can proactively identify emerging fraud indicators by utilizing machine learning and real-time risk scoring.
Tip #3: Adopt the same payment fraud detection solution across all banking channels
Sophisticated fraudsters are always looking to launch attacks at the weakest, most exposed points in your payments ecosystem. This can be customer facing devices such as ATMs, third party APIs, the payments switch or the back-end fraud system itself. Focusing payment fraud detection and prevention efforts disproportionately on one channel may also lead to vulnerabilities elsewhere.
Although there is no silver bullet to fraud, you may want to consider adopting a cross functional network-based solution that provides a centralized view into the real-time performance of self-service devices, networks, legacy systems, third party applications, host authorization points and switches. These powerful solutions are designed to pick up on system compromises in real-time, while also analyzing the risk of every individual payment transaction flowing across the enterprise-wide payments infrastructure – regardless of which channel they originated from.
If you are interested in more tips on network-based fraud solutions, machine learning and real-time transaction risk scoring to help defend against ghoulish payment fraud attacks, treat yourself by downloading the free whitepaper titled, “Machine Learning and Risk Scoring for Real-time Payment Fraud Detection and Prevention”.
Additionally, If you are looking for card-present and card-not-present fraud solutions check cnp fraud prevention solution from INETCO.